Insurance (Personal and Commercial Lines)
Automated Policy Advisory in Insurance
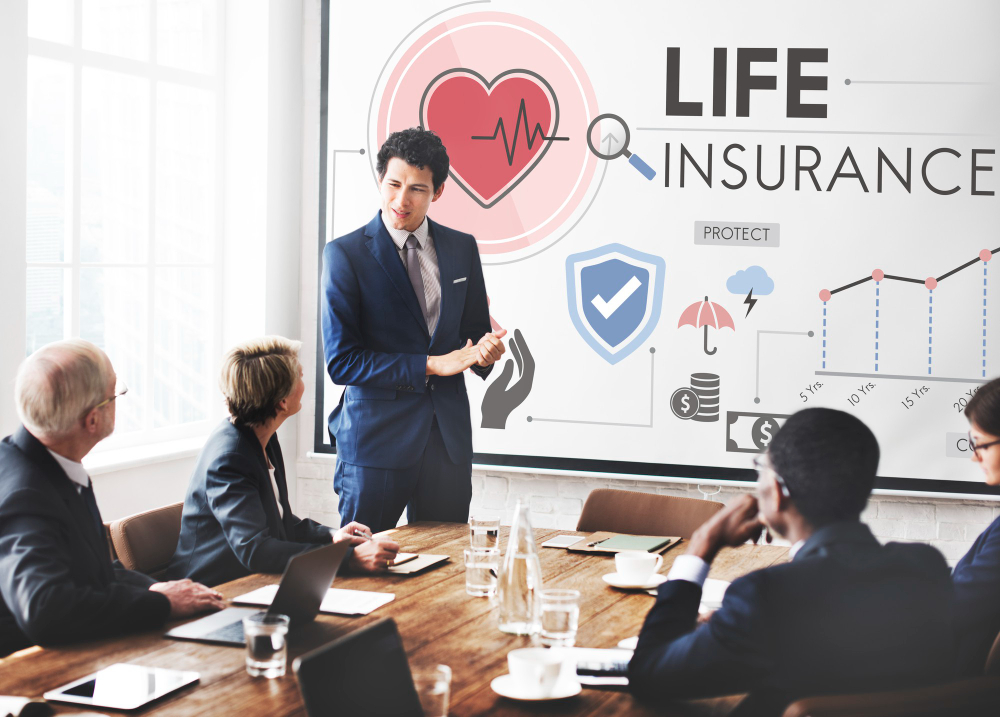
Company
Industry: Insurance (Personal and Commercial Lines)
Number of Employees: 4,000
Year Founded: 1970
Country: Canada
Problem
Customer service representatives frequently struggled to access the latest information on various policy options. They spent significant time flipping through multiple systems, which delayed policy issuance and undermined cross-selling opportunities.
Solution
A RAG chatbot was integrated with the insurer’s policy database, claims data, and regulatory documents. In real time, it generated personalized policy recommendations and detailed explanations, helping representatives expedite customer interactions and improve upsell accuracy.
Result
- Before: Typical call-handling time was 10 minutes
- After: Call-handling time decreased to 5 minutes, a 50% reduction
- Additional Outcome: Cross-selling success rate rose from 12% to 20%, boosting revenue
A large franchisor streamlined operations with Quepasa RAG, centralizing 5,000+ documents into a unified knowledge base. An AI bot provides quick, accurate answers, reducing query resolution time by 75% and improving content accuracy to 95%, enhancing overall service quality.
A fabric wholesaler optimized B2B support with Quepasa RAG, unifying product data and enabling an AI-powered query bot. Response times dropped by 80%, manual lookups were minimized, and sales conversions increased by ~15%.
A dynamic community optimized knowledge sharing with Quepasa RAG, integrating chats, transcripts, and profiles into a searchable system. Search time dropped by 80%, engagement increased by 25%, and redundant questions fell from 50% to 10%.
Visa and legal consultancies streamlined research with Quepasa RAG, a centralized AI-powered legal repository. It reduced research time by 60% and cut follow-up clarifications from 70% to 25%, improving efficiency and client satisfaction.
No-code/API platforms simplify the creation of custom search agents. RAG Platform API enables search customization without coding through an intuitive drag-and-drop interface. As a result, development time is reduced by 90%, and 80% of changes can be made by non-technical users, decreasing IT workload.
A Brazilian telecom company (8,000 employees, founded in 1990) faced high service delays and costs due to inefficient troubleshooting. A RAG-powered mobile app cut repeat site visits by 25 points and reduced customer downtime by 40%, enhancing service efficiency.
A Canadian insurance company (4,000 employees, founded in 1970) faced slow policy access and missed cross-selling opportunities. A RAG chatbot cut call-handling time by 50% and increased cross-selling success from 12% to 20%, driving efficiency and revenue growth.
A US private hospital network (2,000 employees, founded in 1995) faced inefficiencies due to scattered patient data. Implementing a RAG-powered EHR integration cut documentation time by 60%, improving workflow and patient care.
A UK e-commerce company (5,000 employees, founded in 2008) struggled with high support demand and slow response times. A RAG-based chatbot, integrated with its knowledge base, reduced response time by 83% and increased customer satisfaction from 70% to 92%.
A German industrial equipment manufacturer (3,500 employees, founded in 1980) faced slow technician training and production downtimes. A RAG-powered system cut onboarding time by 33% and reduced downtime by 20%, improving efficiency and cost savings.
A Singapore investment firm (1,200 employees, founded in 2002) faced slow risk assessments and missed market opportunities. A RAG-powered assistant cut reporting time by 80% and sped up trade execution by 30%, improving decision-making.
An Australian ed-tech startup (250 employees, founded in 2015) struggled with student engagement and slow feedback. A RAG-driven tutoring tool boosted retention by 25 points and improved test scores by 15%, enhancing learning outcomes.